Correcting the COVID Record, Part I - Unmasking the Data
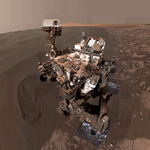
Co-published on Odysee, Publish0x, and Zirkels.
When the COVID pandemic began, there was a large emphasis from governments and the medical community to wear masks as a way to stop the spread of the coronavirus. Some governments even went as far as to implement mask mandates and punish people for not wearing masks via fines. Of course, there were many people who resisted and opposed such mandates because they were too authoritarian.
There were two camps in the mask debate. One claimed that masks provided a protective effect by trapping respiratory droplets whenever a person sneezes or coughs. The other claimed that masks do not significantly reduce the spread of COVID. Anthony Fauci infamously flip-flopped on masking, initially telling Americans to not use masks before going the complete opposite direction.
How An Unproven Narrative Proliferates
The "Masks help reduce the spread of COVID" narrative dominated the collective mindset. My guess as to how the narrative become so dominant is due to two reasons: (1) It was spread and repeated by 'authoritative' figures like Fauci and (2) Biological plausibility. The first is easy to explain. The majority of people do not have the medical knowledge and expertise that the 'authoritative' figures possess. As a result, they tend to trust these figures with little question and fall into the Appeal to Authority trap.
The second reason is more complex, but also plays a major role why so many believed that masking works. Biological plausibility is a concept in epidemiology where if a relationship between the putative cause and outcome is consistent with biological knowledge, then that biological process must be the cause for the relationship. The explanation as to how masks supposedly mitigate the spread of COVID is easily understandable and plausible to the normal person.
However, biological plausibility is actually one of the weakest pieces of evidence for causality. My old article about blue-light blocking glasses is a good example of this. Yes, exposure to blue light inhibits the release of melatonin, a hormone that helps with sleep. You would think if you wear blue-light blocking glasses, you will have better quality sleep. However, the study that I cited in my article found inconclusive evidence.
English epidemiologist Dr. Austin Bradford Hill suggested against using plausibility. His reasoning is that biological and medical knowledge constantly evolves. What may be "odd" or "impossible" today can be explainable tomorrow. He cited the relationship between chimney sweeps and excess cancer in the 18th century as an example. The concept of cancer back then was foreign, but just because it was foreign does not mean cancer does not exist. Limiting one's conclusions to what is biologically plausible introduces bias.
What Does the Data Actually Say?
This brings the main focus of this article: a mask study from Jefferson et al. (2023) from the Cochrane Library. This is a multi-hundred page review that looks at the effects of masking, using N95 respirators, hand washing, and gargling on respiratory virus cases along with several other secondary outcomes like deaths and hospitalizations.
Why this study, in particular? Other than that it was very recently published, it only includes individual-level randomized control trials, cluster RCTs, and quasi-RCTs in the meta-analysis. These types of studies have one of the highest levels of evidence compared to case control, cohort, and cross sectional studies.
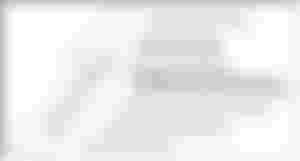
For simplicity's sake, I will only focus on the medical/surgical masks vs. no masks and the N95 vs. surgical masks comparisons. In the first analysis, the study looked at cases of influenza/COVID-like illness, lab-confirmed influenza or COVID illness, and lab-confirmed other respiratory illnesses. Results showed no statistical significance between the mask and no mask groups. The risk ratios for flu/COVID-like illness and lab-confirmed flu or COVID were close to 1, meaning that wearing masks did not reduce nor increase the risk of catching the diseases. Although the risk ratio for lab-confirmed 'others' was a low 0.58, the confidence interval is extremely large hence why the p-value is way above 0.05.
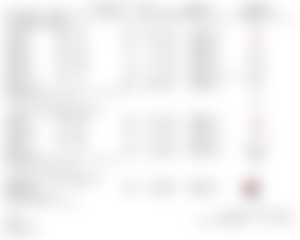
As for N95 masks vs. surgical masks, the results were also statistically insignificant. While there were 0.70 and 0.82 risk ratios for clinical respiratory illness and flu-like illness, respectively, the p-values were above 0.05. With lab-confirmed influenza, the risk ratio was a 1.10 with a p-value of 0.37. The study also found that there was significantly more discomfort from wearing N95 masks than surgical masks.
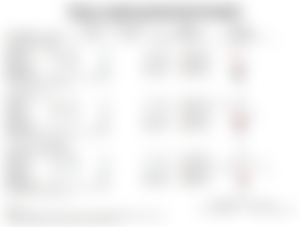
Understanding Type I and Type II Error
In order to understand what these results say, we need to first understand the concepts of Type I and II errors. Type I errors are commonly referred to as 'false positive' error, i.e. "analysis shows masks have a protective effect when there actually is not". Type I error is usually set a 5% and if the p-value falls above 0.05, then you have to conclude statistical insignificance. Sometimes, scientists will reduce the Type I error to a smaller percentage for reasons like small sample sizes. Proving statistical significance with a lower Type I error is more difficult.
Type II error is referred to as 'false negative' error, i.e. "analysis shows masks do not have a protective effect when there actually is one". Type II error has a direct relationship with something called statistical power. Mathematically, statistical power equals to 1 minus Type II error.
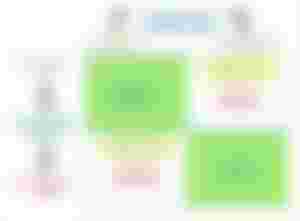
In the context of this study, Type II error is more pertinent as the analyses concluded no statistical significance. A quick and easy way to guess whether there is high statistical power is the sample size. Increasing the sample size is among the most practical and used tactic to increase statistical power. In the tables above, the sample sizes are in the thousands which would suggest high power. As a result, the probability of the study concluding a false negative when it comes to masks is low.
Closing Thoughts: Always Check and Verify
Just because something is biologically plausible and it 'makes sense', it does not necessarily mean it is true. It is very easy to fall into that herd mentality and blindly trust the 'experts'. However, no matter what, it is always a good thing to investigate for yourself even if you are not an expert on that particular subject matter.
This also puts into perspective the government and Big Tech's crackdown on so-called 'medical misinformation'. A lot of people got either suspended or banned on social media platforms for expressing skepticism towards the recommended COVID measures at the time, whether it's masking, the lockdowns, or the vaccines. That is completely counterproductive and harmful to science which is a constantly evolving field. You do not "Trust the Science™". Science is never "settled". To quote Dr. Austin Bradford Hill:
In short, the association we observe may be one new to science or medicine and we must not dismiss it too light-heartedly as just too odd. As Sherlock Holmes advised Dr. Watson, ‘when you have eliminated the impossible, whatever remains, however improbable, must be the truth.’
Stay tuned for Part II.
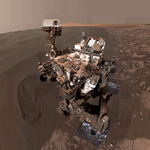