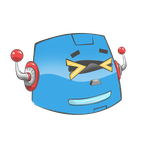
Working With Data: The Power of AI and Machine Learning
In late 2021, Facebook announced it was changing its name to Meta.
And honestly, that is an incredibly brilliant marketing idea for its targeted audience (the one massive negative for it is ‘Meta’ means ‘dead’ in Hebrew. Unfortunately, the number of people who associate ‘meta’ with something positive far outweighs this).
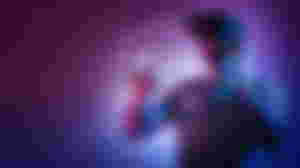
You see, the “Metaverse” is NOT new… it’s been quietly evolving for some time.
Let’s revisit some history!..We are here to put the puzzle together in technical terms but the scope and intent is to show what’s behind and we try to layout in layman terms in the next 3 articles to present the Metaverse , lets try with ;
Clueless to Knowledgeable ( background )
Later in My next article , We will Uncover Beginners Metaverse Understanding
Beginners Metaverse Profiting Ideas
Beginners Metaverse Mastery
Do note we mention the word Beginners ,as the Metaverse will progress beyond and we cannot speculate How it will evolve further . .Do follow us as we will bring you on the Metverse Journey
And honestly, that is an incredibly brilliant marketing idea for its targeted audience (the one massive negative for it is ‘Meta’ means ‘dead’ in Hebrew. Unfortunately, the number of people who associate ‘meta’ with something positive far outweighs this).
You see, the “Metaverse” is NOT new… it’s been quietly evolving for some time.
Let’s revisit some history!
By now it’s no secret that digital data arrives by the truckload and that it can be worth its weight in gold. But that knowledge isn’t half as important as understanding how you can tame the data beast and then wring out every drop of its value.
Naturally, creative and resourceful people in one place or another are always finding new processes and applications that’ll make better use of their data. But we’ll explore some of today’s dominant data utilization trends and leave predicting tomorrow’s technology for the pundits.
Exactly What Is Data?
Before priming our understanding of what’s available to help us work productively with data, it’s a good idea to first define exactly what data is. Sure, we saw plenty of great individual examples in the previous chapter (Managing Data Storage), including the huge volumes of performance and status information produced by digital components of complex systems like cars. But that’s not the same as a definition.
So then let’s define it. Data, for our purposes, is any digital information that is generated by, or used for, your compute operations. That will include log messages produced by a compute device, weather information relayed through remote sensors to a server, digital imaging files (like CT, tomography and ultrasound scans), and the numbers you enter into a spreadsheet. And everything in between.
Which brings us to big data — another one of those buzz phrases that get thrown around a lot, often without accompanying context or clarity.
How would you defined big data?
If you answered that big data describes data sets that come in volumes higher than traditional data software and hardware solutions are capable of handling, then you’re on target. Although we could add one or two secondary characteristics.
The complexity of a data set, for instance, is also something that could force you to consider big data solutions. And sets of data that must be consumed and analyzed while in motion (streaming data ) are also often better addressed using big data tools.
It’s worth mentioning that big data workloads will often seek to solve large scale predictive analytics or behavior analytics problems. ……. Essentially, big data is nothing more than large data sets. These large data sets are increasingly common online, seeing as everything online is easy to measure and document. If you think about a company like Google, it has immense data sets that it works from, describing the search history of billions of users.
But even a standard website that gets 1,000 visitors a day will work with huge amounts of information. A website will naturally record each of those visits and will also store data about each one — such as the country of origin, and the length of time spent on the site.
In a few weeks, this data will likely crash a lot of spreadsheet software!
The reason that big data is featured in so many discussions is that it is very difficult to handle. Making sense of such huge amounts of information requires a lot of smart math, while simply storing and handling that kind of data requires a lot of storage and computational power.
But the potential value of big data is also absolutely huge. Big data provides patterns and insights that you simply can’t get by observing a few users. This is essentially how machine learning works — by looking for patterns in massive data sets. The difference is that this is being leveraged in a slightly different way.
Predictive Modelling
Predictive modelling is a process that involves data mining and probability to forecast potential future outcomes. A model is created using a number of “predictors.” Predictors are variables that are thought to influence future results.
Once data is collected for those predictors, a statistical model can be created. That might use a simple linear equation, or it might use complex neural networks. Either way, statistical analysis can then be used in order to make predictions about how things are likely to go in future.
Collecting Big Data
If you want to start collecting data for your business, there are a wide number of plugins and tools you can use to do so.
You should find that a lot of tools, such as Google Analytics, will allow you to export massive amounts of data in order to work on.
You can then choose to use this information yourself, or to outsource it to a data science organization that can use that information to provide valuable, useful insights.
Such problems are common within domains like healthcare, Internet of Things (IoC), and information technology.
With that out of the way, we can now get to work understanding how — and why — all that data is being used.
Virtual Reality and Augmented Reality
Why? Plain old reality suddenly not good enough for you?
Well yes, in some cases, plain old reality really isn’t good enough. At least if you have a strong interest in engaging in experiences that are difficult or impossible under normal conditions.
A virtual reality (VR) device lets you immerse yourself in an non-existent environment. The most common examples of currently available VR technology feature some kind of headset that projects visual images in front of your eyes while tracking your head movements and, in some cases, the way you’re moving other parts of your body.
The visual images will adapt to your physical movements, giving you the sensation that you’re actually within and manipulating the virtual projection.
VR has obvious potential applications in educational, healthcare, research, and military fields. The ability to simulate distant, prohibitively expensive, or theoretical environments can make training more realistic and immediate than would be otherwise possible.
VR technologies have been arriving — and then disappearing — for decades already.
For the most part, they’ve focused on providing immersive gaming and entertainment environments.
But they’ve never really caught on in a big way beyond the niche product level. This might be partly due to high prices, and because some people experienced forms of motion sickness and disorientation.
But maybe — just maybe — <insert the current year here> will finally be the year VR hits the big time.
But where VR can leverage data in a really meaningful way is when, rather than blocking out your physical surroundings, the virtual environment is overlaid on top of your actual field of vision. Imagine you’re a technician working on electrical switching hardware under a sidewalk.
You’re wearing goggles that let you see the equipment in front of you, but that also project text and icons clearly identifying labels for each part and that show you where a replacement part should go and how it’s connected.
This is augmented reality.
I’m sure you can easily imagine how powerful this kind of dynamic display could be in the right conditions. Surgeons are able to access a patient’s history or even consult relevant medical literature without having to divert their eyes from the operation.
Military pilots can similarly enjoy “heads up” displays that show them timely status reports describing their own aircraft and broader air traffic conditions without distraction.
So why is Augmented Reality (AR) so important to understand if we’re focusing on the *Metaverse*?
Mainly because it’s one half of the Metaverse!
Before , we branch into Uncover Beginners Metaverse Understanding, we must finish and complete the following, as all such basic introduction to Metaverse ,will require the readers to has some understanding of the following :-
Artificial Intelligence and Machine Learning
Before we go further, we should first take a look at precisely what AI and machine learning actually are. These are two related but also distinct terms, which often get confused. Both will impact on marketing, but in different and unique ways.
AI then is artificial intelligence. That means software and hardware designed to act and appear intelligent. Such software is capable of making meaningful choices, and conducting activities that we would normally consider the remit of humans.
AI comes in two broad flavors. One is weak AI, which is also known as narrow AI. Weak AI is essentially a form of AI that is designed to perform a specific job.
An example of this is the self-driving car.
This form of AI is capable of knowing the positions of countless cars on the road, and being able to respond by steering, accelerating, breaking etc. If you were to watch a self-driving car from the outside, you might think a human were driving. In that way, it does a job that would normally be considered a human role.
BUT at the same time, you can’t speak with a self-driving car and you can’t ask it how it’s feeling. A self-driving car would certainly not pass the Turing Test!
Note: The Turing test is a test designed to measure the effectiveness of an AI. If you talk to an AI on a chat app, and you don’t know that it isn’t human, then it is considered to have “passed the Turing test.”
Another example of weak AI is used when creating bad guys in computer games.
These use programming in order to behave in a human-like manner, and to provide a challenge for the player. However, the code is only useful in the context of the video game, and so it’s not about to turn into Skynet any time soon!
Weak AI might not sound as exciting, but it is being used for a huge range of extremely exciting things — from helping to treat disease, to improving the economy.
Conversely, the type of AI that we often see in science fiction, is what we know as “general AI.” This is AI that doesn’t have just one purpose, but that is designed to do everything that a human might be able to do.
So you could play a word game with this AI, ask it how it’s feeling, or get it to look up something useful.
An example of a general AI is DeepMind, owned by Google. DeepMind is a company that has developed a “neural network,” that employs “general learning algorithms” to learn a huge range of different skills.
Many AIs such as IBM’s Watson are actually pre-programmed. That means that they work using a kind of flow chart, and will answer questions with the same answer every time.
On the other hand, DeepMind is apparently able to think and respond via a “convolutional neural network.”
Certain behaviors and reinforced and encouraged, and these will begin to become more prominent.
This isn’t a perfect simulation of how a human brain works (cognitive behavioral psychology teaches us the importance of having internal dialogues and models for thinking), however it is the closest thing we currently have to a “true” general intelligence.
Machine Learning
Machine learning on the other hand works differently. Machine learning utilizes huge data sets in order to gain surprising and almost frightening capabilities at times.
Machine learning essentially allows a piece of software to be “trained.”
An obvious example of this would be computer vision.
Computer vision describes the ability that some machines have to understand visual information. An example is Google Lens, which can tell you what you’re pointing your phone’s camera at, whether that’s a type of flower, or a product you can buy in stores.
Computer vision is necessary for self-driving cars to successfully navigate their environments, and it’s used by apps like Snapchat which use filters to change people’s faces.
How do these work?
By looking at thousands and thousands of pictures of every type of object. While the machine learning algorithm will never understand what it is looking at, it can look for patterns in the data which will then be useful to identify those objects in future.
For example, it might notice that faces are typically oval in shape, with a dark patch of hair on top. It then knows that if it sees an oval shape with a dark patch at the top, it’s possibly looking at a face.
Machine learning has HUGE potential in just about every field. In future, it can be used to diagnose disease more accurately than a human doctor, to advise on financial decisions, to identify fraudulent bank transfers, and much more.
All of this has HUGE potential implications for internet marketing, and that’s what we’ll be exploring in the following chapters.
As a rule, computers are even better at performing dull, repetitive tasks over and over again than bored teenagers pretending to do homework. And they don’t need parents to clean up after them.
The trick with computers is to cleverly string lots of dull, repetitive tasks together so that they can approximate intelligent and useful behavior.
The prize at the end of that road is called automation. Or, in other words, a state where computers can be confidently left alone to perform complex and useful tasks without supervision.
In many ways, we’ve been living in an age of sophisticated computer automation for decades. Domains as diverse as security monitoring, urban traffic control, book manufacturing, and heavy industry are already being handled with little or no human supervision.
But artificial intelligence (AI) seeks to go beyond relatively simple repetition to train computers to think for themselves — and thereby efficiently solve far more difficult problems.
Great idea. Somewhat harder to achieve in the real world.
What can AI actually do?
Understanding how effective AI can be will depend on what you expect it to do.
Can you design software to search for and flag a handful of suspicious financial transactions from among the millions of credit card transactions a large bank processes?
Yes. Although I’m not quite sure that’s truly AI at work and not just automation.
Can you deploy “intelligent” chatbots on your website to help customers solve their problems without needing actual (and expensive) human interaction?
Yes. In fact, I just had a surprisingly effective conversation with my mobile phone carrier’s chatbot that did quickly solve my problem.
Can the first stages of a rocket you’ve just used to launch a payload into space use AI to guide it to a safe landing on a moving platform in the middle of the ocean?
If you’d ask me, I’d say it’s impossible. But SpaceX went ahead anyway and did it multiple times. Good thing they didn’t ask me.
But can AI reliably make strategic decisions that intelligently account for all the many moving parts and complexity that exist in your industry?
Can an AI-powered machine pass the Turing test (where a human evaluator is unable to be sure whether the machine is also human)?
Perhaps not just yet. And perhaps never.
One tool used in many AI processes is the neural network.
The original neural network consists of the many neurons that carry information about the state of a biological environment to the brain.
Artificial and virtual neural networks are systems for assessing, processing, and responding to the large physical or virtual data sets that feed AI-controlled systems.
Such data can come from cameras or other physical sensors, or from multiple data sources. The processed data can sometimes be used for predictive modeling, where the likelihood of future outcomes are compared.
Exciting stuff, to be sure. But the tools used for some of the most significant accomplishments attributed to artificial intelligence aren’t actually artificial. Nor did they necessarily require all that much intelligence.
For example, Amazon Mechanical Turk (MTurk) is a service that connects client companies with remote freelancing “human intelligence” workers.
The workers will, for what usually amounts to dreadfully low pay, perform “mechanical” tasks like labeling the content of hundreds or thousands of images.
The labeling will cover areas like “is the subject a male or female?” or “is the subject a car or a bus?”
It could be that, over time, services like Mechanical Turk will become less important as improving AI methodologies might one day completely replace the human element for this kind of work.
But in the meantime, MTurk and its competitors are still steaming along at full speed, churning out millions of units of “artificial” artificial intelligence.
One methodology that can help reduce reliance on human intervention is machine learning (ML).
How can machine learning help?
ML works by leveraging various kinds of manual assistance to help achieve greater task automation. An ML system can hopefully “learn” how to manage our tasks by being exposed to existing training data.
Only once the the system has demonstrated sufficient skill at solving the problems you have for it, will it be let loose on “real world” data.
These are some common approaches to training your ML system:
Supervised learning lets the ML software read data sets that include both “problems” (images, for example) and their “solutions” (full labels). By seeing enough of the provided examples, the system should be able to apply its experience to similar problems that arrive without solutions.
Unsupervised learning simply throws raw data without any associated solutions at the system. The goal is for the software to recognize enough patterns in the data to allow it to solve the problems on its own.
Reinforcement learning learns from interactions with its environment. Ideally, the software recognizes and understands positive results and evolves its methodology to reliably and consistently produce similar results.
Deep learning algorithms apply multiple layers of analysis to transform the raw target data. The full, multi-layer process in deep learning is known as the substantial credit assignment path (CAP).
AI in general, and ML in particular, are effective at building tools for tasks like autonomous driving, drug discovery, email filtering, and speech recognition, and for deriving sentiment analysis from massive data sets made up of human communications .
In case you’re wondering just what you’re seeing in the above picture…
Pokemon Go *virtually overlays* the images of a Pokemon in your *real life* (well, your real life as seen by the camera).
Almost like Virtual Reality, right?
Except not.
What you just saw is called *Augmented Reality*.
At the time of this writing, Virtual Reality requires a headset.
But *augmented reality* simply uses your phone and overlays images that would be considered desirable.
And it’s not limited to gaming, either!
Companies are adding augmented reality to their products so people can visually “see” what a purchase might look like:
So why is Augmented Reality (AR) so important to understand if we’re focusing on the *Metaverse*?
Mainly because it’s one half of the Metaverse!
The other half is Virtual Reality.
Virtual Reality (VR) is experienced with a VR headset that entirely envelops your eyes:
Because your field of vision is contained within the headset, you can “see” and “interact” with experiences that are NOT *real life* (aka Virtual, aka Virtual Reality).
Please Note :
The article was originally published On Medium
under a new Publication hoste dby Medium
Metaverse — Clueless to Master …the Story begins .
Thank you ,the next few article will be of short form content, but we will try to include more pictures to make it more interesting
Disclaimer : No claim is made to the exclusive right to use “metaverse” apart from general descriptive terms as per article as shown above ,,
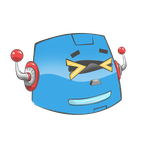