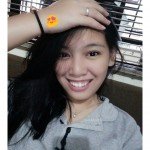
Predictive analytics is a form of data analytics that uses historical data and analytics techniques including statistical modeling and machine learning to make predictions about future outcomes. Predictive analytics is a science that can produce potential insights with a high degree of accuracy. Any company can now use past and current data to anticipate patterns and behaviors milliseconds, days, or years in the future with the aid of advanced predictive analytics software and models.
According to a 2017 study published by Zion Market Research, predictive analytics has gained the support of a wide variety of organizations, with a global market expected to reach approximately $10.95 billion by 2022, expanding at a compound annual growth rate (CAGR) of about 21% between 2016 and 2022.
At work, predictive analytics
Predictive analytics uses a variety of tools and technology, such as big data, data processing, predictive modeling, machine learning, and various mathematical processes. Predictive analytics is a method of sifting through current and historical data to identify patterns and forecast events and conditions that may occur at a particular time based on defined parameters.
Organizations may use predictive analytics to identify and exploit trends in data in order to spot threats and opportunities. Models can be created to discover associations between different behavior variables, for example. Such models allow for the evaluation of a set of conditions' promise or risk, guiding informed decision-making through a variety of supply chain and procurement events.
See "7 secrets of predictive analytics performance" for advice on how to efficiently leverage the power of predictive analytics.
Predictive analytics' advantages
Predictive analytics improves the accuracy and reliability of looking into the future over previous methods. As a result, it will be able to assist adopters in finding ways to save and earn money. Predictive models are often used by retailers to forecast inventory needs, monitor delivery schedules, and customize store layouts in order to optimize sales. Predictive analytics is widely used by airlines to adjust ticket prices based on previous travel patterns. Hotels, restaurants, and other players in the hospitality industry may use the technology to predict the number of guests expected on any given night in order to optimize occupancy and revenue.
Organizations can produce new consumer feedback or sales, as well as encourage cross-sell opportunities, by optimizing marketing strategies with predictive analytics. Businesses may use predictive models to help them attract, maintain, and nurture their most valuable customers.
Predictive analytics may also be used to spot and stop different forms of illegal activity before it causes significant harm. An company can detect unusual activities such as credit card fraud, corporate surveillance, and cyberattacks by using predictive analytics to study user habits and acts.
Examples of predictive analytics
Predictive analytics is now used in a nearly limitless number of forms for businesses. Finance, healthcare, retailing, hospitality, pharmaceuticals, automotive, aerospace, and manufacturing are among the industries that benefit from the technology.
Here are some examples of how businesses are using predictive analytics:
Predict the effect of specific maintenance operations on aircraft reliability, fuel consumption, availability, and uptime in the aerospace industry.
Automotive: Incorporate component durability and failure records into future vehicle production plans. Driver behavior is being studied in order to create improved driver assistance systems and, potentially, self-driving cars.
Long-term price and demand ratios are forecasted for energy. Determine how weather, equipment failure, legislation, and other factors affect service costs.
Develop credit risk models for financial services. Financial industry patterns are forecasted. Estimate how new policies, rules, and legislation will affect companies and markets.
Predict the location and rate of system failures in manufacturing. Optimize raw material deliveries based on potential demand projections.
Use crime pattern data to identify areas that can need extra security at certain times of the year.
Retail: Track an online customer in real time to see whether offering more product details or offers would improve the probability of a successful purchase.
Tools for predictive analytics
Predictive analytics software provides users with deep, real-time insights into a virtually infinite number of business activities. Predictions based on an analysis of data collected over time can be used to predict different forms of actions and trends, such as how to allocate resources at specific times, when to replenish stock, or the best time to launch a marketing campaign.
Almost all predictive analytics users depend on tools created by one or more third-party developers. Many of these resources are specifically designed to meet the needs of particular businesses and agencies. The following are some of the most well-known predictive analytics tools and service providers: Acxiom, IBM, Information Builders, Microsoft, SAP, SAS Institute, Tableau Software, Teradata, TIBCO Software.
Models of predictive analytics
Predictive analytics is built on models, which are the templates that enable users to transform historical and current data into actionable insights, resulting in positive long-term outcomes. Predictive models come in a variety of shapes and sizes.
Customer Lifetime Value Model: Identify consumers who are most likely to buy more goods and services in the future.
Customer Segmentation Model: Consumers are grouped together based on common characteristics and buying habits.
Predictive Maintenance Model: Estimate the likelihood of critical equipment failing.
Model for Quality Assurance: Identify and prevent defects to avoid consumer dissatisfaction and additional costs when delivering goods or services.
Techniques for predictive modeling
Users of models have access to a nearly limitless number of predictive modeling techniques. While several techniques are specific to specific products and services, a core of generic techniques, such as decision trees, regression, and even neural networks, are now commonly supported across a broad variety of predictive analytics platforms.
One of the most widely used techniques, decision trees, rely on a graphical, tree-shaped diagram to decide a course of action or to display a statistical likelihood. The branching method may also show all of the possible outcomes of a given decision, as well as how one choice can lead to the next.
Banking, saving, and other finance-related models often employ regression techniques. Users can use regression to forecast asset values and understand relationships between variables like commodities and stock prices.
Neural networks are at the cutting edge of predictive analytics techniques, since they are algorithms that simulate the way the human mind operates to classify underlying associations within a data set.
Algorithms for predictive analytics
Adopters of predictive analytics have easy access to a variety of mathematical, data-mining, and machine-learning algorithms that can be used in predictive analysis models. Algorithms are typically created to solve a particular business problem or set of problems, improve upon an existing algorithm, or provide a unique functionality.
Customer segmentation, group identification, and other social-related functions, for example, are well-suited to clustering algorithms. Classification algorithms are often used to increase consumer satisfaction or build a recommendation framework. When creating a credit score system or predicting the outcome of several time-driven events, a regression algorithm is usually chosen.
In the field of healthcare, predictive analytics is becoming increasingly popular.
For one simple reason, healthcare companies have become some of the most enthusiastic predictive analytics adopters.
Predictive analytics is used by healthcare organizations in a variety of ways, including intelligently allocating facility resources based on historical patterns, managing personnel schedules, recognizing patients at risk of a costly near-term readmission, and applying intelligence to pharmaceutical and supply procurement and management.
Over half of healthcare executives (57 percent) at organizations already using predictive analytics believe the technology will allow them to save 15% or more of their total budget over the next five years, according to a 2017 Society of Actuaries report on healthcare industry trends in predictive analytics. A further 26% expected a 25 percent or greater savings.
The majority of healthcare executives (89 percent) work for companies that use predictive analytics now or intend to do so within the next five years, according to the survey. A whopping 93 percent of healthcare executives believe predictive analytics is critical to their company's potential success.
What is the best way for a company to get started with predictive analytics?
While getting started with predictive analytics isn't easy, it's a challenge that almost any company can manage as long as it's dedicated to the strategy and willing to put in the time and money required to get the project off the ground. Starting with a small-scale pilot project in a crucial market environment is a great way to keep startup costs down while reducing the amount of time it takes for financial incentives to start rolling in. Once a model is implemented, it typically needs little maintenance as it continues to provide actionable insights for several years.
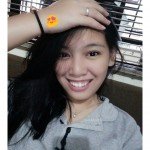
Yes . only knowledge of events and reasoning make prediction possible.