Quality Control and Anomaly Detection in Manufacturing Processes using MLOps.
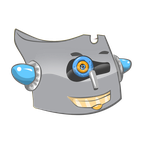
In the world of manufacturing, quality control and anomaly detection are critical aspects that determine the success and efficiency of production lines. Quality control ensures that the products manufactured meet the requirements, standards and specifications, while anomaly detection helps in identifying any deviations from the norm that could potentially lead to defects or malfunctions in the final product.
Traditionally, these processes have been manual and time-consuming, often leading to delays and increased costs. However, with the advent of Machine Learning Operations (MLOps), a new paradigm has emerged that promises to revolutionize these critical aspects of manufacturing.
MLOps, a compound of “Machine Learning” and “Operations”, is a practice for collaboration and communication between data scientists and operations professionals to help manage the production ML lifecycle. MLOps looks to increase automation and improve the quality of production ML while also focusing on business and regulatory requirements.
Applications of MLOps in Manufacturing
In the context of manufacturing, MLOps can be used to automate quality control and anomaly detection processes. This is achieved by training machine learning models on historical manufacturing data, which includes information about the manufacturing process and the corresponding quality outcomes. These models can then predict the quality of new products and detect any anomalies in the manufacturing process in real time.
For quality control, MLOps can be used to develop predictive models that can forecast the quality of a product based on the parameters of the manufacturing process. This allows for proactive quality control, where potential issues can be identified and rectified before the final product is produced.
for anomaly detection, machine learning models can be trained to understand the normal behaviour of a manufacturing process. Any deviations from this norm can be flagged as anomalies. This can be particularly useful in identifying issues that may not be immediately apparent to human operators, such as subtle changes in machine performance or environmental conditions.
Benefits of MLOps in Manufacturing
The use of MLOps in manufacturing processes offers several benefits. Firstly, it increases the efficiency of quality control and anomaly detection processes by automating them. This not only reduces the time and effort required but also minimizes the chances of human error.
Secondly, MLOps allows for proactive quality control and anomaly detection. By predicting the quality outcomes and detecting anomalies in real-time, it enables manufacturers to rectify issues before they escalate, thereby reducing waste and saving costs.
Thirdly, MLOps can help in improving the accuracy of quality control and anomaly detection. Machine Learning models can identify complex patterns and relationships in the data that may be missed by traditional methods. This leads to more accurate predictions and detections, thereby enhancing the overall quality of the manufacturing process.
Lastly, MLOps facilitates continuous learning and improvement. The machine learning models can be continuously updated and improved based on new data and feedback, leading to better performance over time.
Conclusion
In conclusion, MLOps presents a promising solution to enhance quality control and anomaly detection in manufacturing processes. By leveraging machine learning and automation, not only increases efficiency but also improves accuracy and facilitates continuous improvement. As the manufacturing industry continues to evolve, the adoption of MLOps is set to become increasingly prevalent, paving the way for smarter, more efficient and higher-quality manufacturing processes.
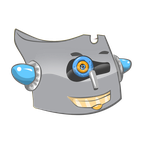