Artificial Intelligence and Machine Learning: Transforming the Future

Artificial Intelligence (AI) and Machine Learning (ML) are at the forefront of the technological revolution, reshaping industries and redefining the way we live, work, and interact with the world. While often used interchangeably, these two terms refer to distinct but interrelated fields. Understanding their differences and the potential they hold is crucial to appreciating their impact on modern society.
Defining AI and ML
Artificial Intelligence is a broad field encompassing any technique that enables computers to mimic human intelligence. This includes problem-solving, understanding natural language, recognizing patterns, and making decisions. AI is characterized by its ability to perform tasks that typically require human intelligence, such as visual perception, speech recognition, decision-making, and language translation.
Machine Learning, a subset of AI, refers to the development of algorithms that allow computers to learn from and make predictions based on data. Instead of being explicitly programmed to perform a task, ML algorithms use statistical techniques to identify patterns in data and improve their performance over time. The more data these algorithms process, the more accurate their predictions become.
How AI and ML Work
AI systems are designed to solve complex problems by emulating human cognitive processes. These systems rely on large datasets, advanced algorithms, and computational power. Key components of AI include:
1. Natural Language Processing (NLP): Enables machines to understand and respond to human language.
2. Computer Vision: Allows machines to interpret and make decisions based on visual inputs.
3. Robotics: Involves the creation of intelligent robots that can perform tasks autonomously.
4. Expert Systems:Use predefined rules to simulate human decision-making in specific domains.
ML, on the other hand, operates through a cycle of training, testing, and refining algorithms. Key types of ML include:
1. Supervised Learning: Algorithms are trained on labeled data, meaning the input data is paired with the correct output. Common applications include image classification and spam detection.
2. Unsupervised Learning: Algorithms find patterns in unlabeled data. This approach is used for clustering and association tasks, such as customer segmentation.
3. Reinforcement Learning: Algorithms learn by interacting with an environment and receiving feedback through rewards or penalties. This method is used in robotics and game playing.
Applications of AI and ML
The applications of AI and ML are vast and growing. Some key areas include:
1. Healthcare:AI-driven diagnostics, personalized medicine, and robotic surgeries are transforming patient care. ML algorithms can analyze medical images with high accuracy, predict disease outbreaks, and assist in drug discovery.
2. Finance: AI and ML are used for fraud detection, risk management, algorithmic trading, and personalized financial advice. They help in analyzing large volumes of data to detect anomalies and predict market trends.
3. Retail: AI enhances customer experiences through personalized recommendations, chatbots, and inventory management. ML models predict consumer behavior and optimize supply chains.
4. Transportation: Autonomous vehicles and traffic management systems rely heavily on AI and ML to improve safety and efficiency. Predictive maintenance of vehicles is another crucial application.
5. Entertainment: Streaming services use ML to recommend content, while AI-generated music and art are emerging creative fields.
Ethical Considerations and Challenges
Despite their benefits, AI and ML pose significant ethical and practical challenges. Issues such as data privacy, algorithmic bias, and job displacement need careful consideration. Ensuring that AI systems are transparent, fair, and accountable is crucial to their responsible deployment.
1. Data Privacy: The vast amounts of data required for AI and ML raise concerns about user privacy and data security. Ensuring that personal data is protected is paramount.
2. Bias and Fairness: AI systems can perpetuate or even exacerbate existing biases if the data they are trained on is biased. It is essential to develop algorithms that are fair and unbiased.
3. Job Displacement: Automation driven by AI could displace many jobs, necessitating strategies for workforce retraining and economic adaptation.
4. Accountability: As AI systems become more autonomous, determining accountability for their actions becomes challenging. Clear regulations and ethical guidelines are needed.
The Future of AI and ML
The future of AI and ML is promising, with advancements in areas like quantum computing and neuromorphic engineering potentially revolutionizing the field. AI's integration with other technologies such as the Internet of Things (IoT) and 5G will further expand its capabilities.
Continued investment in AI research, coupled with ethical considerations, will be crucial to harnessing the full potential of AI and ML. As these technologies evolve, they hold the promise of solving some of humanity's most pressing challenges, from climate change to healthcare, ushering in an era of unprecedented innovation and progress.
In conclusion, AI and ML are not just buzzwords but powerful tools transforming various sectors. Their potential is vast, but so are the challenges they present. A balanced approach that emphasizes innovation while addressing ethical concerns will be key to realizing their benefits for all of humanity.
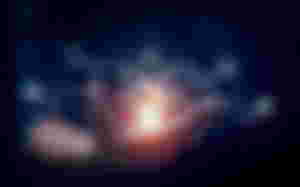
