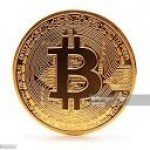
This article is a rundown of wordings characterized in a straightforward way that empowers simple review.
Don't hesitate to utilize this rundown to invigorate on watchwords and definitions on your way to a meeting.
Or then again maybe you are as of now a drop Data Scientist or Machine learning master, yet you might want to return to your establishments. Or on the other hand you need to sit back.
Whichever way before the finish of this article, you will be preapared to comprehend the language of our yet to be overlords.
Key Terms
1. Neuron: In the realm of profound learning and AI, a neuron can be portrayed as a preparing unit that is one of the basic structure squares of a neural organization.
2. Loads: These are the strength of interneuron associations. They are the basic component in the putting away of information inside neural organizations.
3. Neural Network: An assortment of neurons (preparing units) in a way that empowers the maintenance of information. They can likewise be depicted as equal appropriated processors.
4. AI: Is the study of the usage of PC calculations or directions that are coordinated to gain from information, where the calculation is used for an undertaking related with the information it gained from.
5. Profound learning: This is a region of AI where calculations influence the usage of a few layers of neural organizations to remove more extravagant highlights from input information. Instances of profound learning methods are Convolution Neural Networks(CNN) and Recurrent Neural Networks(RNN)
6. Administered learning: This is the preparation of an AI calculation with information commented on with names. The explanation of information is ordinarily given by a specialist framework, for example, a human or outside framework. The assignment of arrangement is an illustration of an administered learning task.
7. Unaided learning: Algorithms intended to handle this sort of learning make them arrange qualities incorporated into them. These calculations self put together information dependent on examples distinguished in information without the inclusion of a specialist framework.
8. Semi-regulated learning: Machine learning calculations that are semi-managed comprise of both unlabeled and named preparing information. The recurrence of marked information in the appropriation of the preparation dataset is normally on a more modest scope in contrast with unlabeled preparing information.
9. Fortification learning: This is a kind of Machine learning procedure that includes characterized programs that are alluded to as specialists. These specialists are set in a climate and are administered by the thought of the expansion of remunerations through cooperations with the climate. The specialists are intended to expect to gather rewards where conceivable. There is likewise the type of negative prizes or punishments. The specialist task is to improve its administering framework to gather awards after some time and dodge punishments.
10. Bunch Learning: This is a strategy for the introduction of preparing information to an AI calculation's organization. Preparing information amassed is taken care of to the AI calculation at the same time, and changes are made to the predisposition and the loads of the organization being prepared once all preparation information have been taken care of forward.
11. Web based Learning: This strategy for introducing preparing information to the organization is completed gradually. The preparation information is part into bunches alluded to as scaled down groups, and once a smaller than usual clump has been taken care of through the organization, an update is made to the organization's loads and inclination, at that point another little cluster is then taken care of forward. This cycle is rehashed until all little bunches are gone through the organization.
12. Speculation: Machine learning calculations and models can be arranged dependent on the estimation of their exhibition on concealed information.
13. Model: This can be depicted as a numerical portrayal of the summed up example saw in a dataset.
14. Example based learning (memory-based learning): An AI framework can sum up dependent on perception of examples inside preparing information introduced to the calculation during the preparation stage. These examples (cases) can be used to improve the speculation of the AI calculation by computing some comparability estimation scores dependent on new occurrences introduced during testing with the cases utilized in preparing. The calculation is said to make forecasts on new cases dependent on past examples of perceptions during preparing.
15. Model-based learning: An elective strategy for actualizing a framework that can sum up instead of example based learning, is to utilize a produce a model dependent on a dataset and utilize the model to handle errands, for example, forecasts.
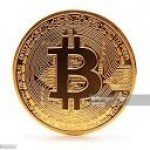
Artificial intelligence is present in many industries. ChatGPT itself is a revolution. The normal thing is that IA is starting to be present in marketing. If anyone is interested in this topic, I recommend this post: https://gamerseo.com/blog/ai-influencer-know-everything-about-virtual-influencers-marketing/